- Home
- / Insights
- / FTI Journal
Who’s Minding Your Artificial Intelligence?
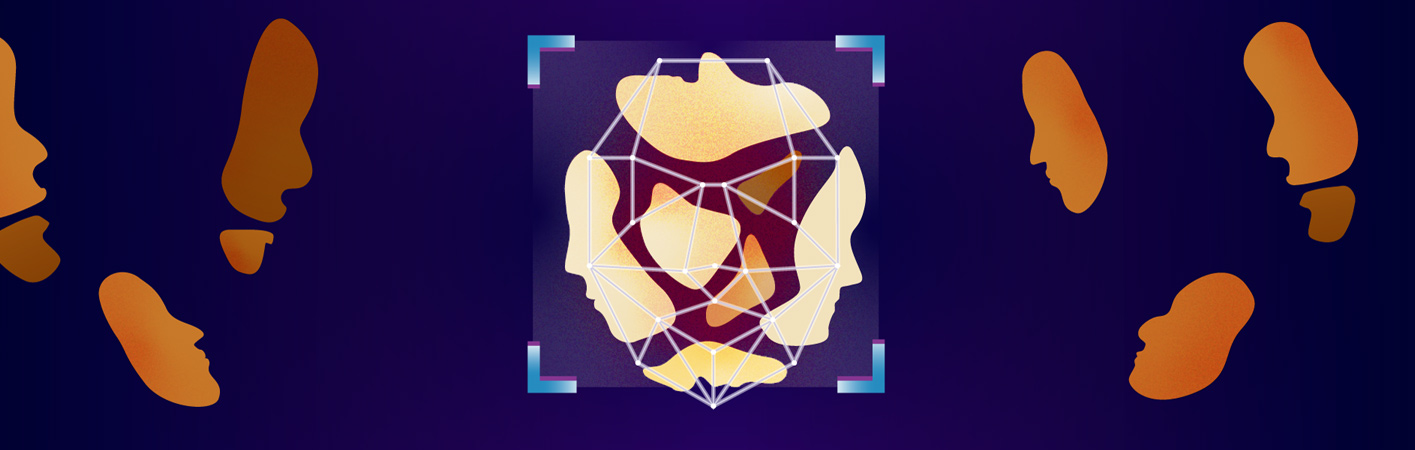
-
December 09, 2021
-
Once the stuff of futuristic sci-fi novels and movies, artificial intelligence (AI) is now so deeply ingrained in society that we almost take it for granted. AI is the brain that powers machine learning (ML) applications that streamline so many business functions. ML apps perform repetitive back-office tasks, for instance, forecast sales, route customer service requests and generate detailed medical diagnoses for doctors.
Supported by sophisticated algorithms in the AI, the applications learn and improve performance as they operate over time. It is truly the future realized.
But there may well be a hidden glitch in the system.
As businesses increasingly rely on ML to make decisions that impact individuals — in critical areas such as hiring, credit lending, health care and criminal justice, to name four — concerns about bias within AI are on the rise. Questions about fairness and discrimination are raging across social media and news outlets as various groups of people and individuals are excluded from positive results.
Regulators and legislators within the United States and European Union are directing businesses to take a hard look at the issue, known as an “accountability gap,” and mitigate it.
Who is to blame? It is not necessarily the businesses themselves. In fact, company leaders may not even be aware of the issue until they come up against the flames of social media or run afoul of regulators.
While companies regularly target specific demographics for legitimate business purposes, the intention is not to replicate, reinforce or amplify existing biases in their ML models. The issue stems from data collected or used by the AI itself, which is a human construct. And because humans are inherently biased, your AI may contain biased information as well.
The results generated by your AI algorithms can imperil your company’s reputation or trigger unwanted regulatory scrutiny, because the more your ML models run with bias, the more they reinforce that bias.
Identifying the sample data in your AI that is causing biased results and mitigating the issue can be challenging. This is science, after all. But it is a must. Closing the accountability gap is not only a regulatory and legal imperative, but also an ethical one with societal implications.
AI and Human Bias
To understand how your application’s algorithm might go astray, it helps to take a quick look at the way AI operates and its relationship to the human mind.
AI and Machine Learning Typically defined as the ability of a machine to perform cognitive functions we associate with the human mind, examples of technologies (machines) that utilize AI are robotics and autonomous vehicles, computer vision, computer language, and virtual agents.
According to theory, humans have two systems of thinking. System 1 has to do with automatic, quick thinking that operates with little to no voluntary control. This is our “gut instinct.” System 2 involves more deliberate effort and is linked to action and choice. Drawing on information and experience, system 2 enables us to make predictions and decisions. This is the mode of AI.
AI algorithms mimic system 2 thinking by detecting patterns in large data sets to make predictions and recommendations. They enable ML models to become “smarter” — or more effective at their jobs — as they acquire more data and experiences.
Your ML models might be hard at work right now slicing the tranche of customers most likely to buy your next product, for instance. Or they could be deciding who should be approved for a loan or be invited for a job interview. They could be engaged in a chatbot discussion with one of your customers.
AI algorithms are not programmed for bias. (In fact, AI can help us identify our own biases.) But when they draw from a data set, they reflect the information and experiences of the humans who programmed them. Thus, it is easy to see how bias can get baked into AI.
Who Is in Your AI Data?
Obviously you cannot let AI off the hook simply because it does not “know” any better. You want to get out ahead of an issue.
What is Bias? As defined by Psychology Today, a bias is a cognitive shortcut that can result in judgments that lead to “a tendency, inclination or prejudice toward or against something or someone.”
One starting place is to look at “sample bias.” This occurs when a data set used to train the model does not reflect the environment in which a model will run. Say you want to target individuals for a credit card offer based on median income of U.S. households. But your AI is populated with financial data collected only from individuals in Manhattan, the heart of New York City and one of the wealthiest locales in the United States. Your results, or model, will be skewed, omitting potential customers in less-affluent cities where the median income is lower.
Other types of AI bias include “prejudicial bias,” which occurs when the training data is influenced by stereotypes or prejudice found in the data population. “Algorithm bias” is bias and variance that is inherently included in modeling. And “measurement bias” produces results from faulty measurements.
You can take a tactical approach to fixing issues as they arise. But better to think strategically and make an independent AI assessment a regular part of periodic risk assessments and standard governance practices — just as you would with any other risk scenario, such as cybersecurity or regulatory compliance. With an interdisciplinary approach that includes applying advanced statistical methods and an end-to-end framework review, you can determine how AI is being used across your full organization. An assessment can identify unintended biases, drive strategic remedial plans and provide technical solutions to support monitoring and reporting.
As AI continues to further advance business functionality, its potential to improve our lives while serving humankind seem limitless. That is heady stuff. If we get it right, we all profit.
© Copyright 2021. The views expressed herein are those of the author(s) and not necessarily the views of FTI Consulting, Inc., its management, its subsidiaries, its affiliates, or its other professionals.
About The Journal
The FTI Journal publication offers deep and engaging insights to contextualize the issues that matter, and explores topics that will impact the risks your business faces and its reputation.
Published
December 09, 2021
Key Contacts
Senior Managing Director
Senior Managing Director
Senior Managing Director
Managing Director
Senior Director